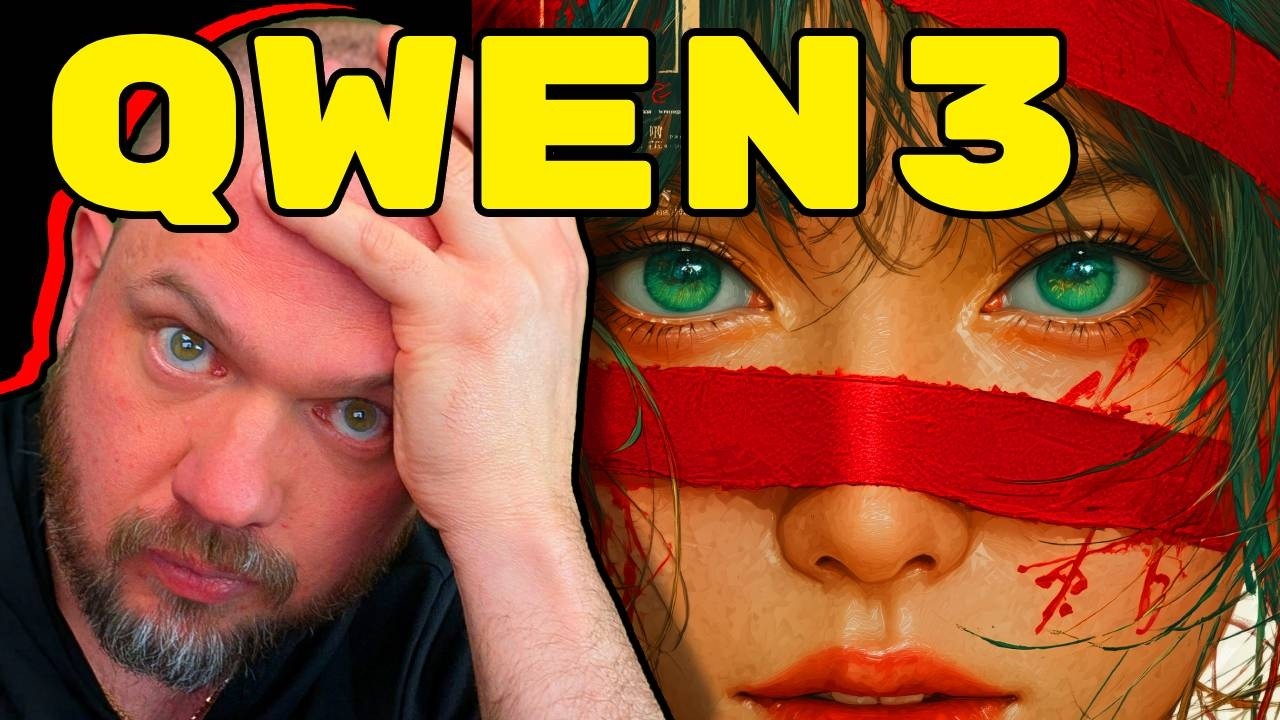
The AI landscape has just experienced another seismic shift with the unexpected arrival of Qwen3, a powerful new open-source AI model from China that's challenging established industry leaders. While many were anticipating a new release from Deepseek, Qwen3 has emerged as a formidable competitor to top-tier models like Gemini 2.5 Pro, OpenAI's GPT models, and other leading AI systems.
Understanding Qwen3's Model Architecture
Qwen3 represents a family of models with varying capabilities and sizes. The flagship model, Qwen3-235B-A22B, uses a mixture of experts (MoE) architecture—a sophisticated approach where different specialized parts of the model are activated depending on the specific task. This means that despite having 235 billion total parameters, only about 22 billion (the "A22B" in its name) are activated for any given query, making it more computationally efficient than traditional dense models.
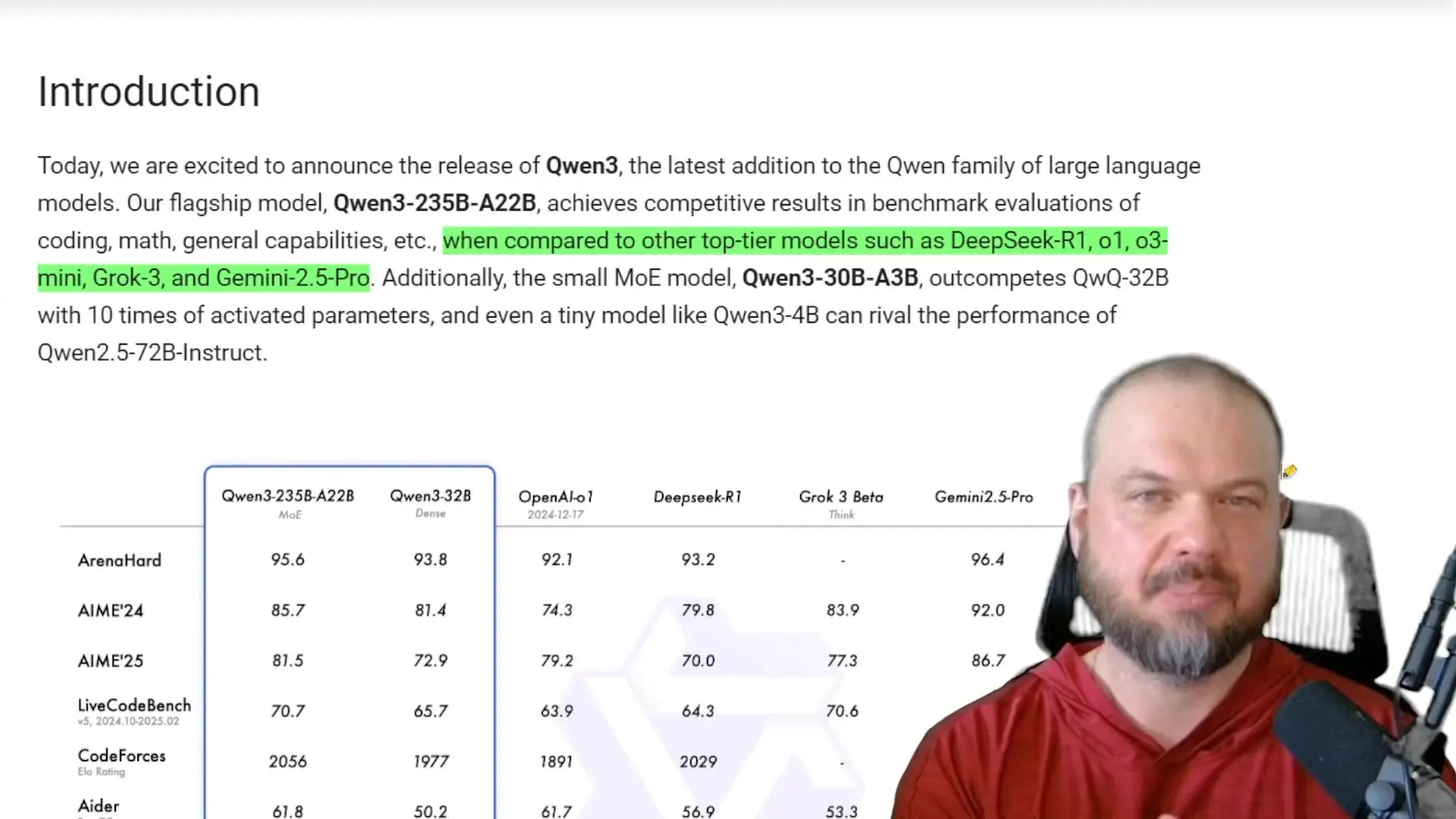
In addition to the flagship MoE model, Qwen3 also includes six dense models (where all parameters are used for every query) ranging from 32 billion parameters down to 6 billion. These smaller models offer more deployment flexibility for different computational environments and use cases.
Qwen3's Unique Dual Thinking Modes: A Game-Changing Feature
One of Qwen3's most innovative features is its support for both "thinking" and "non-thinking" modes. This dual-mode capability allows users to choose between quick responses or more thorough, reasoned answers depending on their needs.
- Thinking Mode: Engages the model's reasoning capabilities, processing information more thoroughly before producing an answer. Performance improves significantly as the thinking budget (measured in tokens) increases.
- Non-Thinking Mode: Provides fast, direct responses without extended reasoning, ideal for straightforward queries where speed is prioritized over depth.
Testing shows that Qwen3's performance on complex tasks like mathematical reasoning (AIME 24/25) and coding challenges improves dramatically when allowed to "think" with higher token budgets. For instance, performance on AIME benchmarks rises from around 50% with non-thinking mode to approximately 85% when given a 16,000-token thinking budget.
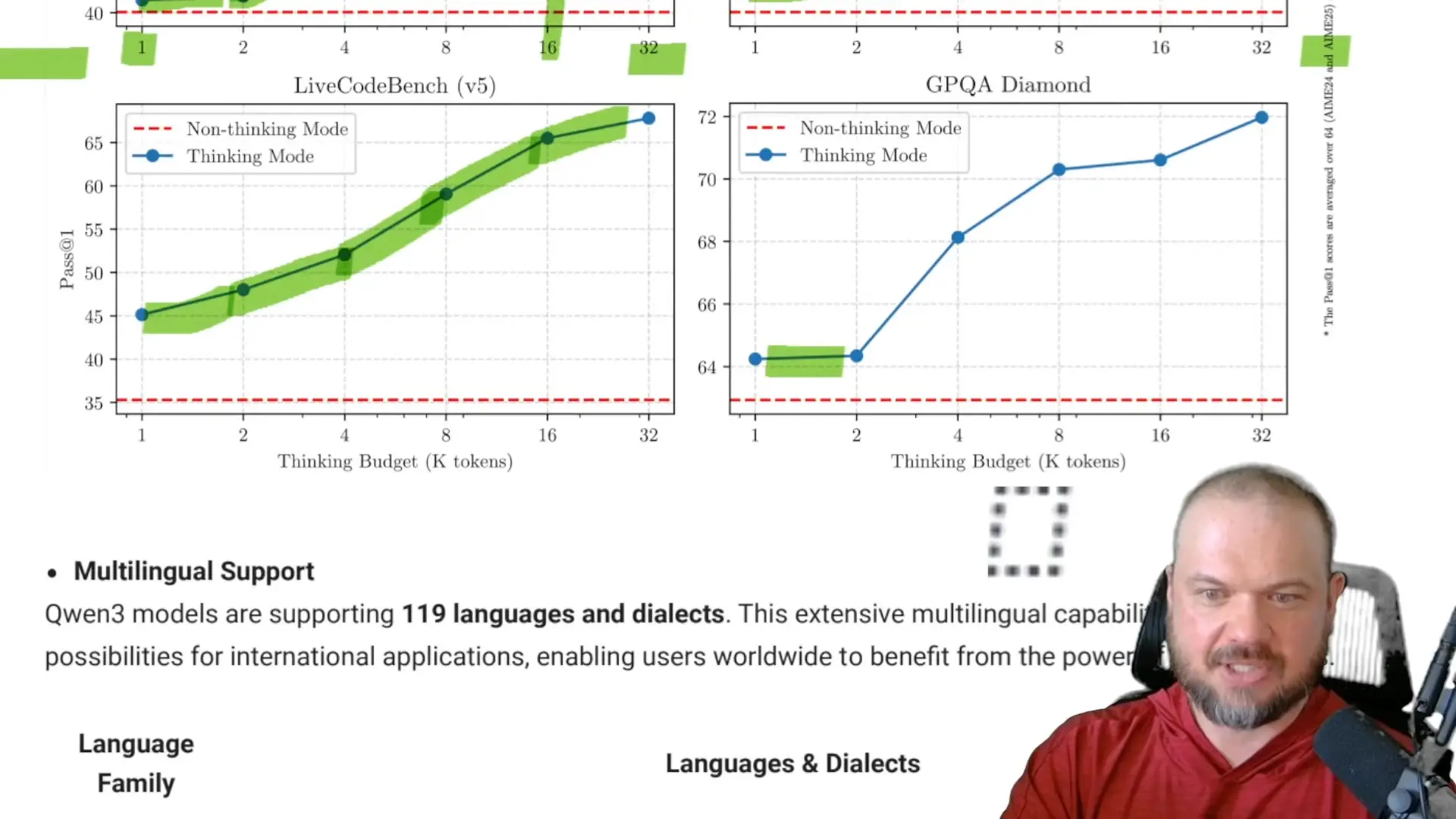
Benchmark Performance: How Qwen3 Stacks Up Against Industry Leaders
Qwen3 demonstrates impressive performance across multiple benchmarks, often outperforming OpenAI's models and competing closely with Google's Gemini 2.5 Pro:
- On AIME 24 (a high-end mathematical competition benchmark), Qwen3 places between Gemini 2.5 Pro and OpenAI's O3 Mini
- For LiveCodeBench, Qwen3 actually outperforms Gemini 2.5 Pro
- On CodeForces, it beats both Gemini 2.5 Pro and OpenAI's O3 Mini
- Across other benchmarks, it remains highly competitive with top commercial models
While benchmarks alone don't tell the complete story of a model's real-world utility, Qwen3's consistent strong showing across diverse evaluation metrics suggests it has achieved a level of general capability previously seen only in the most advanced proprietary models.
Multilingual and Technical Capabilities
Qwen3 supports an impressive 119 languages and dialects, making it one of the most linguistically diverse AI models available. This extensive language support positions it well for global applications and research.
The model also demonstrates enhanced capabilities in several technical domains:
- Improved coding abilities across multiple programming languages
- Enhanced agentic capabilities for more autonomous task completion
- Strengthened support for Model Context Protocol (MCP) for interacting with various software tools
- Strong performance on STEM topics including mathematics, engineering, and scientific reasoning
The Massive Training Dataset Behind Qwen3
Qwen3's impressive capabilities stem from its extensive training process. The model was pre-trained on nearly twice the data of its predecessor, Qwen 2.5, with approximately 35 trillion tokens from diverse sources including web content and PDF documents.
Interestingly, the Qwen team leveraged their previous models to improve the current generation:
- Qwen 2.5VL (vision-language model) was used to extract text from visual documents
- Qwen 2.5 helped improve the quality of extracted content
- Qwen 2.5 Math and Qwen 2.5 Coder generated synthetic training data for specialized domains
This approach demonstrates how each generation of AI models can bootstrap the development of more capable successors—a virtuous cycle of improvement that accelerates AI advancement.
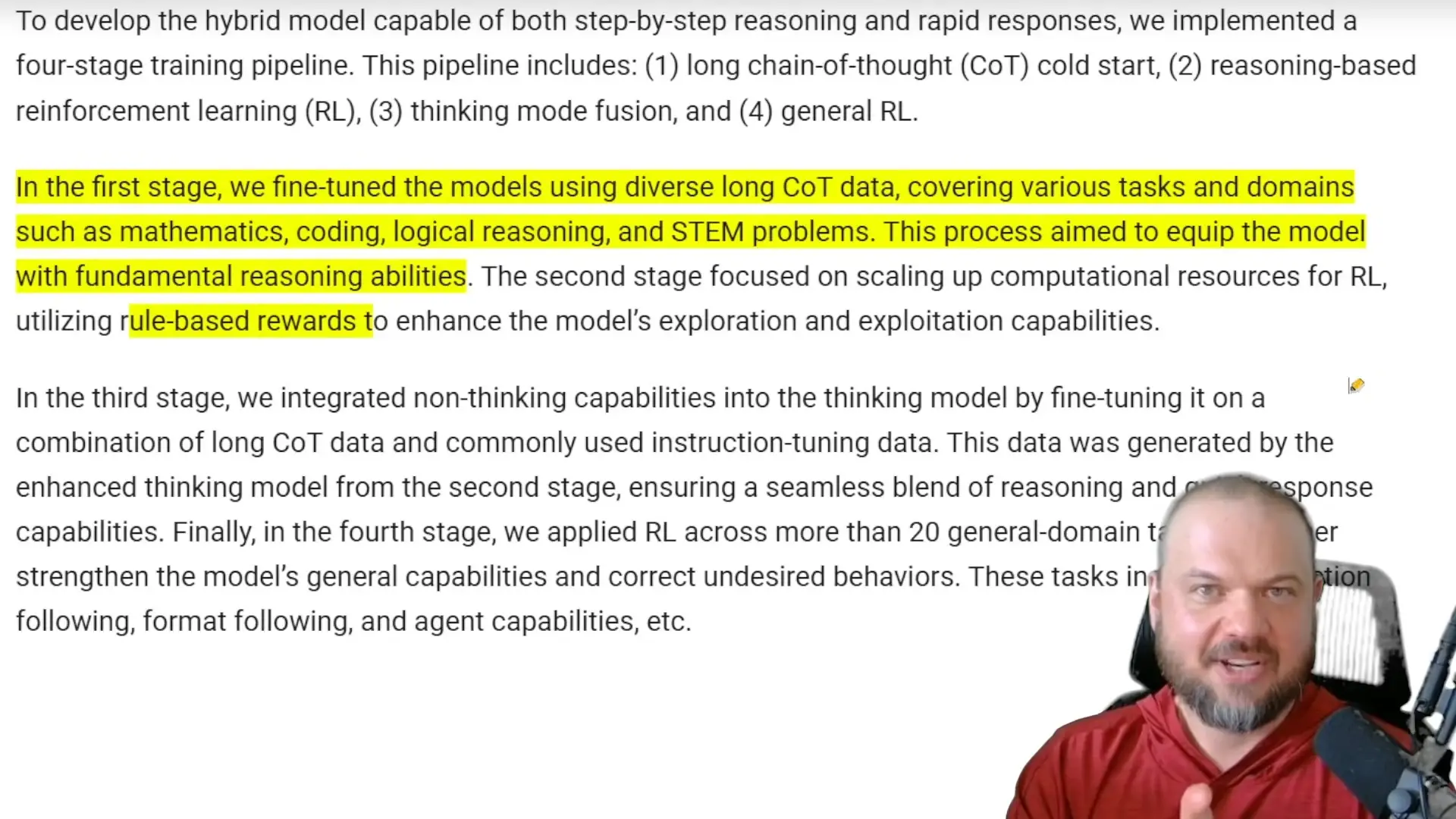
The Multi-Stage Training Process
Qwen3's development followed a meticulous multi-stage process that contributed to its exceptional capabilities:
- Base Pre-training: The initial stage involved pre-training on over 30 trillion tokens with a context length of 4,000 tokens, establishing basic language skills and general knowledge.
- Knowledge Enhancement: The second stage increased the proportion of knowledge-intensive data (STEM, mathematics, coding, reasoning tasks), with an additional 5 trillion tokens of training.
- Context Extension: The final pre-training stage used high-quality long-context data to extend the model's context window to 32,000 tokens.
- Post-training Refinement: This phase included multiple stages of specialized training to enhance reasoning capabilities.
Post-Training Stages
- Long Chain-of-Thought Cold Start: Providing examples to kickstart the model's reasoning abilities
- Reading Reasoning Reinforcement Learning: Positive reinforcement of correct reasoning patterns
- Thinking Mode Fusion: Integration of thinking and non-thinking modes
- General Reinforcement Learning: Broad improvement across all capabilities
For the lightweight models, the team employed strong-to-weak distillation—using the outputs of larger models as synthetic training data for smaller variants. This technique creates faster, more efficient models that retain much of the capability of their larger counterparts.
The Open-Source Advantage: Why Qwen3 Matters for AI Research
Perhaps Qwen3's most significant contribution to the AI landscape is its open-source nature. By releasing not only the trained models but also detailed information about their architecture and training process, the Qwen team is enabling researchers worldwide to build upon their work.
All models are available on popular AI repositories including HuggingFace, ModelScope, and Kaggle, making them accessible to researchers, developers, and organizations globally. This open approach contrasts with the increasingly closed nature of some commercial AI systems and could accelerate innovation across the field.
The developers have hinted that Qwen3 contains "intriguing features" not documented in the model cards that could open new avenues for both research and product development. As the community explores these capabilities, we're likely to see novel applications and further advancements built on the Qwen3 foundation.
Implications for the AI Industry
Qwen3's emergence as a top-tier open-source model has several important implications for the AI industry:
- Increased competition for commercial AI providers like OpenAI and Google
- Democratization of access to state-of-the-art AI capabilities
- Potential acceleration of AI research through open collaboration
- Growing importance of Chinese contributions to global AI development
- New opportunities for applications that benefit from the thinking/non-thinking mode flexibility
Conclusion: A New Contender in the AI Race
Qwen3 represents a significant milestone in AI development—an open-source model family that genuinely competes with the best proprietary systems from tech giants. Its innovative architecture, dual thinking modes, and impressive multilingual capabilities make it a versatile tool for a wide range of applications.
As researchers and developers explore Qwen3's full potential, we're likely to see new use cases and capabilities emerge. The model's release reinforces the vital role of open-source development in advancing AI technology and ensuring its benefits are widely accessible.
Whether Qwen3 will ultimately disrupt the dominance of models like GPT-4 and Gemini remains to be seen, but its strong performance and innovative features certainly make it a contender worth watching closely in the rapidly evolving AI landscape.
Let's Watch!
Qwen3 AI Model: The Unexpected Breakthrough Challenging GPT and Gemini
Ready to enhance your neural network?
Access our quantum knowledge cores and upgrade your programming abilities.
Initialize Training Sequence